Empowering Big Data and Artificial Intelligence through Web Scraping
- Raquell Silva
- Oct 2, 2024
- 7 min read
Updated: Feb 13
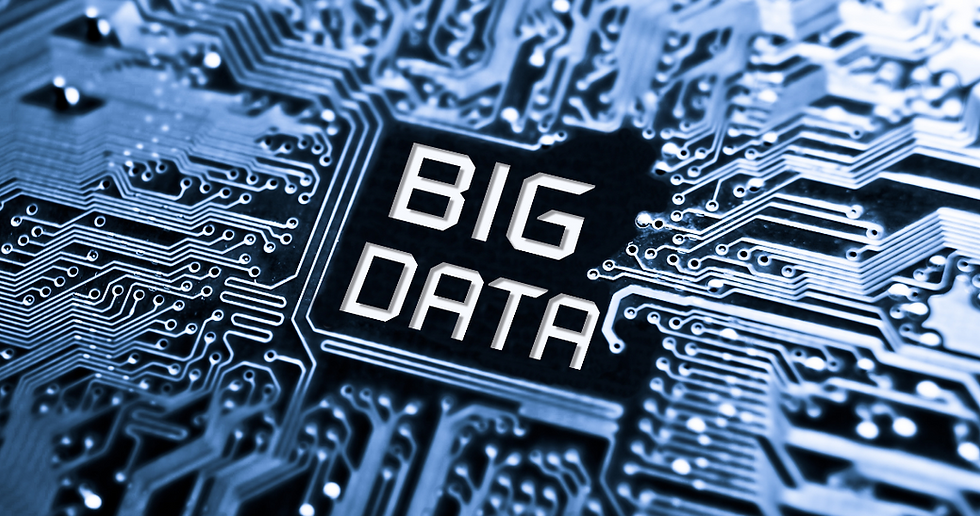
You’ve probably been hearing these two terms a lot lately: Big Data and Artificial Intelligence (AI). Together, they represent monumental shifts in how businesses approach problems and seek solutions. But how exactly do they intersect, and what role does web scraping play in this convergence? In this article, we will explain this relationship and explore what exactly AI needs to be fed in order to learn.
Where Does AI Get Its Data?
Companies have been using AI to launch new solutions, optimize decision-making, improve customer experience, and reduce operational costs. But that is not possible without Big Data, as it plays a crucial role in AI, especially in Machine Learning (ML) models. These models require vast amounts of data to train on, learn from, and make predictions or decisions. The more high-quality data a model has, the better its performance tends to be. However, the vastness of data needed by AI models often poses a significant challenge: access to large datasets. Most companies struggle with amassing this requisite volume of data, especially these data are from external sources such as the Internet.
This is where web scraping comes into play. Web scraping is the number one step to empower any machine learning system. It all starts with collecting the data. Web scraping provides a solution to the problem of data insufficiency by extracting large amounts of relevant data from the web, effectively “feeding” the AI models. Without this method, many businesses would be unable to leverage the full power of AI, simply due to a lack of raw material – the data.
Web scraping feeds the data reservoirs, which, through data mining, uncover actionable insights. These insights then feed AI algorithms, leading to intelligent business strategies and automation.
Let’s sum up:
The output of web scraping provides the raw data for the big data process.
Once this data is structured and stored in big data systems, it’s ready for data mining processes to extract patterns and insights.
The results from data mining then become the foundation for training machine learning models.
How to Improve the Data to Feed AI?
Understanding how to refine and optimize this data becomes paramount to ensure that the AI systems are fed the right kind of information. Here are 5 strategies to enhance the quality of data you introduce to your AI, ensuring it not only performs optimally but also delivers reliable and actionable insights.
Feed more data
Just as humans learn from experiences, AI learns from data. The more data it’s exposed to, the better it learns. Large datasets often encompass a broader range of scenarios, allowing AI systems to understand various situations, outliers, or anomalies. Therefore, the more accurate data you feed the AI model, the more accurate the result.
High-Quality Data Collection
Ensure that the dataset captured is diverse, from various scenarios, cultures, geographies, and situations. Biases in data can lead to inaccuracies. Moreover, removes noise and irrelevant data points and handles missing values appropriately, either by imputation or removal.
Continual Data Collection
Systems and behaviors evolve, so continually collect new data to keep the model relevant. As more data becomes available or the environment changes, regularly update and retrain models. Take note, some old data might no longer be relevant or might mislead the model. Periodically review and prune your dataset.
Feature Engineering
Feature engineering is the process of selecting, modifying, or creating new pieces of information (features) from raw data to improve the performance of a machine learning model. Therefore, identify and use only the most relevant features to reduce the model’s complexity and training time. Transform the data into a format or structure that makes it easier for the model to understand. Techniques like PCA (Principal Component Analysis) can be beneficial.
Collaboration and Expertise
Engage experts from different domains to get diverse perspectives on the data. A finance expert might view data differently than a software engineer or a sociologist. Combining these views can offer a richer understanding.
Who Should Handle Web Scraping to Enhance Your AI?
Hiring a professional web scraping company can be beneficial in several ways, as these companies specialize in extracting large volumes of data from the web, ensuring that data is accurate and relevant. There are other benefits associated with working with professionals:
Expertise: Professional web scraping companies possess specialized knowledge and expertise in the domain. This means they are adept at navigating the myriad challenges associated with data extraction, including handling different website structures, evading potential blockades, and managing requests efficiently. Their deep understanding of scraping ensures that the data extracted is of high quality and meets the specific requirements of the AI model in use.
Scalability: They have the infrastructure to scrape data from multiple sources simultaneously, ensuring vast amounts of data in a shorter time frame.
Compliance: Professional scraping companies are aware of the legal boundaries and will ensure that data extraction respects all regulations and terms of service.
Clean and Structured Data: They not only extract data but can also provide it in a structured and usable format, reducing the preprocessing workload.
Final thoughts
Empowering AI is not just about algorithms and computing power. At its core, it’s about ensuring it has the right data to make informed, accurate, and ethical decisions. As we usher in an era increasingly dominated by AI and machine learning, understanding and managing its primary fuel – data – becomes paramount. For businesses seeking to be at the forefront of innovation, mastering data collection techniques or having the right web scraping partners is not just beneficial; it’s essential.
Companies that strategically leverage these tools not only gain a competitive advantage but also innovate in product and service offerings.
Use Case: Enhancing Algorithmic Trading through Web Scraping and AI-Driven Risk Management
With the rise of AI, algorithms have become even more sophisticated, capable of making highly accurate predictions based on big datasets. One of the unsung heroes in this revolution is web scraping, providing real-time data that breathes life into these algorithms.
AI influenced many industries if not all of them. One of them is the financial industry. With the stock market being influenced by various global events, company announcements, and market news, hedge funds and financial institutions have sought ways to harness these vast pools of information. According to research published by Forbes, 43% of AI consumers use the tool for financial advice.
Adoption of AI
Recognizing the need for faster and more accurate predictions, several leading hedge funds turned to AI-driven models. These models could analyze vast amounts of financial data in real-time. The result was a significant increase in prediction accuracy, translating to better investment decisions.
Integration of Web Scraping
To supplement the AI’s data needs, these funds employed web scraping tools. These tools continuously scoured the web, gathering real-time data from various news sources, financial forums, and company announcements, resulting in:
Real-time Analysis: With web scraping, AI models receive real-time updates. For instance, if a major company made an unexpected announcement, the AI system would immediately be aware of it and adjust its trading strategy accordingly.
Holistic Decision Making: Apart from numerical financial data, the AI system could now consider sentiment analysis from financial forums or global event impacts from news sources, leading to a more holistic trading strategy
Enhanced Risk Management: Earlier, sudden market changes often caught traders by surprise. With the AI-web scraping duo, these institutions could foresee potential risks and adjust their portfolios before a significant market dip, significantly reducing losses.
Challenges
Using web scraping to feed AI with data has its challenges: ensuring the continuous, accurate, and reliable extraction of data from the web. Maintaining scraping scripts and ensuring data relevance became a significant concern.
The Proposed Solution
Recognizing the intricate nature of web scraping, especially when its results are directly influencing high-stakes financial decisions, the solution was evident: leverage the expertise of a reputable enterprise-level web scraping company. Here is how the implementation of the solutions took place:
Partner Selection: A thorough vetting process was undertaken to select a web scraping company with a proven track record in serving enterprise-level clients, ensuring they possess the technical capabilities and understand the nuances of the financial sector.
Customized Data Extraction: This company was not just about off-the-shelf solutions. They collaborated closely with the trading entity, understanding specific requirements, target data sources, and desired data formats. This ensured that the AI models would receive precisely the data they required.
Continuous Maintenance and Support: One of the primary benefits of partnering with an enterprise-level provider was the assurance of continuous maintenance. They regularly updated scraping scripts, accounted for website changes, and ensured uninterrupted data flows.
Quality Assurance and Data Integrity: The provider ensured that the data extracted was not only accurate but also cleaned and structured, ready for integration into AI systems. This eliminated the need for additional data processing and validation.
Scalability and Expansion: As the trading entity’s needs evolved, the web scraping company was equipped to scale operations, ensuring that even as more data sources were added or extraction frequencies increased, the system could handle the surge seamlessly.
Outcomes:
By partnering with a top-tier enterprise-level web scraping company, the trading entity was able to navigate the challenges of data extraction effectively. This collaboration not only ensured optimal trading insights but also positioned the entity at the forefront of technology-driven trading, making the most of the symbiotic relationship between AI and web scraping. The results:
Reliability: There was a marked increase in the reliability of data feeds, ensuring that AI models always had up-to-date information.
Efficiency: By outsourcing the intricacies of web scraping, the trading entity could focus more on refining AI models and trading strategies.
Reduced Overheads: By leveraging the expertise of a specialized company, the trading entity saved significantly on in-house resources and infrastructure costs.
Conclusion:
The integration of real-time data extraction with advanced AI algorithms has a profound impact on the financial industry. By doing so, trading entities not only optimize their strategies but also effectively navigate the myriad challenges posed by the digital data deluge. As we’ve seen, partnering with a reputable enterprise-level web scraping company is not just a strategic choice; it’s a crucial step toward ensuring data reliability, efficiency, and reduced overheads. As the financial sector continues its digital evolution, such symbiotic collaborations between AI and data extraction tools will be pivotal in shaping its future, ensuring that trading decisions are both informed and agile in this age of rapid information exchange.
Comments